Understanding AI & Its Risks in Third Party Networks
- 3 days ago
- 6 min read
This blog was inspired by the meeting facilitated by Julie Gaiaschi, CEO & Co-Founder of TPRA, at TPRA’s March 2025 Practitioner Member Roundtable. (To watch the full presentation, TPRA Members can visit our On-Demand Webinars page and navigate to the March 2025 meeting recording.)
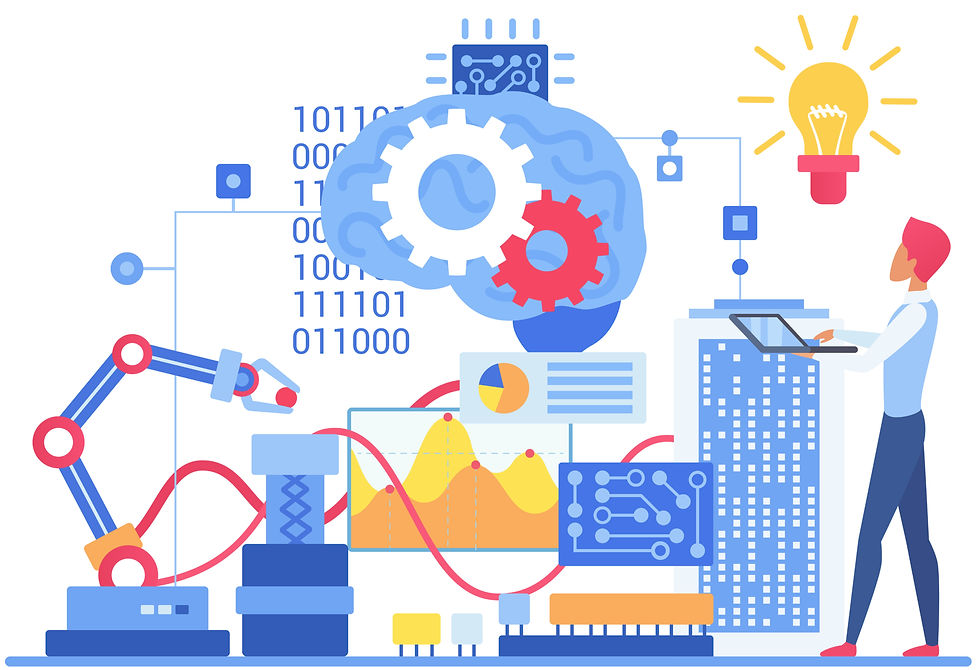
Now-a-days, artificial intelligence (AI) seems to be involved in nearly every type of business activity. It is reshaping business operations by offering increased efficiency, automation, and data-driven insights. Within third party networks, AI driven technologies are influencing how third party risk management (TPRM) practitioners identify and assess risks. This is due to third parties using these AI technologies in critical areas like supply chain management, financial transactions, and cybersecurity. From this increased use of AI, the risks associated with AI are also growing. However, it is important to know that not all AI is the same. In addition, not everything labeled as AI truly fits the definition.
The first step in managing AI risks is to have an understanding of what AI is, and what it is not. According to NIST’s AI Risk Management Framework (RMF), AI is “an engineered or machine-based system that can, for a given set of objectives, generate outputs such as predictions, recommendations, or decisions influencing real or virtual environments. AI systems are designed to operate with varying levels of autonomy.” A custom model is typically not considered AI if it is rule-based or uses simpler statistical methods because the custom model lacks learning or adaptive capabilities.
In this blog we will explore:
Types of AI & Use Cases
Risks Related to AI
Risks Related to AI Metrics
What Should Occur Before Assessing AI Risk
Assessing AI in Third Party Networks
Types of AI & Use Cases
AI systems can be classified based on their functionality, level of intelligence, and application. The list below is not all encompassing, but breaks down some common types of AI.
Expert Systems
Mimic human expertise in specific domains by following a set of programmed rules. Examples include diagnostic tools in medicine and legal analysis systems.
Natural Language Processing (NLP)
Computer Vision
Robotics
Recommendation Systems
Generative AI
Cognitive Computing
Predictive Analytics
Risks Related to AI
Compared to other risks that TPRM practitioners assess, AI technologies have the capability to impact more than just your company. AI technologies pose risks that can negatively impact individuals, groups, organizations, communities, society, the environment, and the planet. Below are some risks that are related to AI, but this is not an exhaustive list. Due to AI technology being so new, risks are still being identified as threat actors use AI for their own personal gain.
AI systems can be trained on data that changes over time, sometimes significantly and unexpectedly, affecting system functionality and trustworthiness.
AI systems and the contexts in which they are deployed are frequently complex, making it difficult to detect and respond to failures when they occur.
AI systems are inherently socio-technical in nature, meaning they are influenced by societal dynamics and human behavior.
Without proper controls, AI systems can amplify, perpetuate, or exacerbate inequitable or undesirable outcomes for individuals and communities.
AI risks or failures that are not well-defined or adequately understood are difficult to measure quantitatively or qualitatively. This means that if you aren't aware of how the AI operates or is being trained, then you may not see a failure or a risk.
Risks Related to AI Metrics
When it comes to AI and understanding how it works, transparency is a key theme. Part of being transparent is thoroughly understanding the metrics that you're using to evaluate AI. There are risks tied to those metrics, and it’s important to recognize how they impact AI performance and decision-making. Some risks related to AI metrics are:
Risk metrics or methodologies used by the organization developing the AI system may not align with the risk metrics or methodologies used by the organization deploying or operating the system. In addition, the organization developing the AI system may not be transparent about the risk metrics or methodologies it used.
Another AI risk metric challenge is the current lack of industry consensus on robust and verifiable measurement methods for risk and trustworthiness, as well as its applicability to different AI use cases.
Approaches for measuring AI decision impacts on a population work if they recognize that contexts matter, that harms may affect varied groups or sub-groups differently, and that communities or other sub-groups who may be harmed are not always direct users of a system.
Measuring risk at an earlier stage in the AI lifecycle may yield different results than measuring risk at a later stage.
While measuring AI risks in a laboratory or a controlled environment may yield important insights pre-deployment, these measurements may differ from risks that emerge in operational, real-world settings.
What Should Occur Before Assessing AI Risk in Third Party Networks?
Before assessing AI risks in third party networks, it is critical to lay the groundwork within your own organization. Establishing clear guidelines and considerations beforehand helps ensure a more effective risk assessment process.
The following steps should be considered:
Create an Acceptable Use Policy to define how AI will be leveraged within the organization, as well as how data will be leveraged within third party AI systems.
Train Employees on what AI is and the acceptable use of AI.
Leverage an AI Framework to inform contracts & assessments (i.e., NIST AI Risk Management Framework is a great example).
Contract for AI - Specify data usage allowed, AI type allowed, ethical considerations, decision-making responsibilities, and data ownership in contracts.
Think through an Exit Strategy for Critical & High risk third parties (consider data retrieval and deletion activities when terminating, model and algorithm ownership, intellectual property rights, data privacy, knowledge transfer, and continuity of operations).
Assessing AI in Third Party Networks
Now that you’ve established AI policies within your own organization, you are ready to assess AI within third party networks. As we assess third-party networks, it's important to recognize that nearly every company today is leveraging AI, whether directly or through their partners. Assessing AI involves similar principles to other information security evaluations, but with distinct challenges. Unique concerns, such as data quality, model interpretability, and the potential for bias, add complexity to AI assessments. Consequently, it’s essential for organizations to prioritize responsible AI development. Developing AI responsibly requires a comprehensive approach that balances innovation with ethical considerations, social impact, and sustainability.
When assessing AI in third party networks, it is important to review the risks related to:
The AI’s Capabilities & Models to determine how effectively and ethically AI systems operate.
Data Quality & Protection to safeguard against ethical, legal, and operational risks, foster trust, and ensure that AI systems operate accurately and securely.
Security & Access Controls to ensure the protection of sensitive data, maintaining model integrity, and ensuring compliance with regulatory standards.
Performance & Reliability to ensure the AI system is operating as intended, adapt to real-world conditions, and deliver dependable outcomes.
Governance & Oversight to ensure the AI system is used responsibly, safely, and effectively. For third party networks, strong governance and oversight help ensure that external partners adhere to the same high standards, preserving the integrity of the organization’s AI ecosystem and protect against external threats.
Conclusion
AI is becoming an integral part of third party networks, and it might be safest to assume that your third parties are using AI in some capacity. This means it is crucial to understand how they are using AI, as well as the potential risks that come from AI and the metrics used to evaluate it. By understanding AI and the risks it poses in third party networks, you can make more informed decisions and strengthen your risk management strategies.
Comentarios